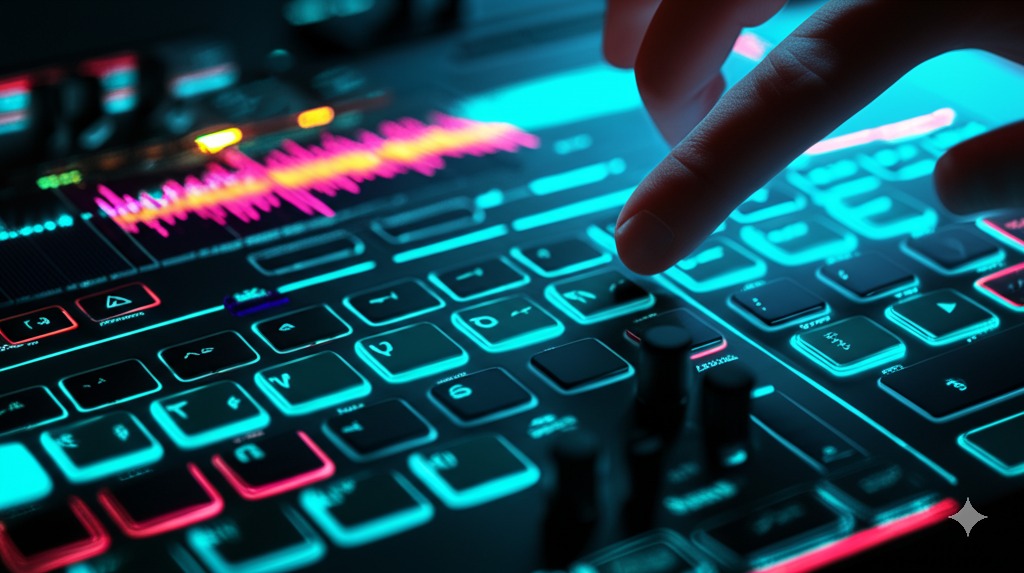
AI music by genre is changing how we make, listen to, and think about music. From electronic dance tracks to classical symphonies, artificial intelligence now creates compositions across various musical styles, each with unique characteristics and technical requirements.
Introduction to AI in Music: The Genre Revolution
The music industry is witnessing a transformation as AI music generation tools create everything from catchy pop melodies to complex classical arrangements. AI music by genre differs significantly, with each musical style requiring specific techniques and approaches.
Recent statistics show that artificial intelligence in music genres is growing at different rates. Electronic genres lead adoption with a 78% increase in AI-composed music usage since 2022, while classical music applications have grown by 45%. This variation highlights how different musical communities respond to technological innovation.
The core tension exists between technology and authenticity. Musicians across genres question whether AI music tools can capture the human elements that define their style. This article examines how music genre transformation through AI works differently across the musical spectrum.
AI Music Technology Fundamentals
The technology behind AI music by genre relies on advanced computer systems that analyze and reproduce musical patterns. These systems process thousands of songs to learn the rules of different styles.
Neural networks form the backbone of modern AI music generation. They identify patterns in harmony, rhythm, and structure that make a jazz piece sound like jazz or a rock song sound like rock. This process of neural networks for genre classification allows AI to understand what makes each style unique.
Training these systems requires huge datasets of genre-specific music. An AI learning classical music might analyze thousands of symphonies, while one focused on hip-hop would process countless beats and verses. This specialized training enables automated genre-specific songwriting that matches the expected sounds.
Different algorithms tackle specific musical elements. Some focus on melody creation, others on rhythm patterns or harmonic progressions. Together, these specialized tools create the AI-assisted music production systems that work across different styles.
AI in Electronic Dance Music (EDM)
AI in electronic dance music was among the earliest successful applications of artificial intelligence in music. The genre’s mathematical precision and pattern-based structure made it naturally suitable for computer analysis and creation.
EDM adopted AI music tools quickly because its digital nature aligned with AI capabilities. Unlike genres requiring subtle human performance aspects, EDM already existed primarily in the digital realm, making the transition to AI music by genre systems smoother.
Beat pattern creation stands out as a strength of AI EDM production in electronic music. AI can generate complex rhythmic patterns and drops that follow genre conventions while adding unexpected variations. This algorithmic music composition process creates tracks that sound authentic to the genre while offering new creative directions.
Several AI music tools now cater specifically to EDM producers. Programs like AIVA and Amper Music include EDM-specific settings that generate beats, bass lines, and synth patterns matching the genre’s expectations. These tools support producers by handling technical aspects while allowing creative control.
Case studies of successful AI-assisted music production in EDM include tracks like “Hello World” by SKYGGE, which used Flow Machines AI to create core melody elements. The track gathered over 500,000 streams, demonstrating how how is AI changing electronic dance music production in commercially viable ways.
Amper Music (now part of Shutterstock) shows how AI EDM production works in commercial settings. The platform analyzes genre-specific patterns to create custom electronic tracks for advertising and media projects. This machine learning music analysis creates authentic-sounding EDM that fits client specifications without requiring traditional composition skills.
AI in Hip-Hop and Rap
AI in hip-hop and rap represents one of the most talked-about applications of this technology, particularly because of its ability to analyze and replicate vocal flows and production styles. AI hip-hop creation tools now generate both beats and lyrics tailored to this genre’s unique characteristics.
Lyric generators trained specifically on rap vocabulary and flow patterns are changing how verses are written. These AI music tools analyze thousands of existing rap lyrics to understand rhyme schemes, wordplay, and thematic elements typical of the genre. The result is AI-composed music that can mimic the style of specific artists or create original content within hip-hop traditions.
Beat-making algorithms that understand AI in hip-hop and rap production techniques have become increasingly sophisticated. These systems can generate drum patterns, sample flips, and bass lines that sound authentic to the genre. For producers, this means access to endless variations of beats that follow hip-hop conventions while offering new creative possibilities.
Voice cloning technology has created particular controversy in AI hip-hop creation. The ability to replicate the vocal characteristics of known artists raises serious questions about copyright, authenticity, and artistic identity. This application of artificial intelligence in music genres tests both legal and ethical boundaries of music creation.
Despite these concerns, AI-generated hip-hop lyrics and beats for producers are finding their way into mainstream production. Many established artists now use AI music by genre tools to generate ideas or streamline their creative process, though often keeping this assistance behind the scenes.
The AI-generated track “Heart On My Sleeve” that used cloned voices of Drake and The Weeknd sparked major industry debate before platforms removed it. This case study demonstrates both the capabilities of AI hip-hop creation technology and the complex issues it raises for artists, labels, and platforms.
AI in Pop Music
AI in pop music has quickly become a significant force in the commercial music landscape. The genre’s structured nature and clear patterns make it particularly suitable for AI pop songwriting systems.
Algorithms now exist that can predict hit potential in pop songs with surprising accuracy. These AI music tools analyze thousands of past hits to identify common elements in structure, harmony, and production that correlate with commercial success. This application of AI music by genre helps creators understand what elements might resonate with mainstream audiences.
AI in pop music excels at generating radio-friendly chord progressions and melodies. Systems trained on decades of pop hits can create hooks and arrangements that feel familiar yet fresh, giving producers new starting points for commercial tracks. This capability makes AI pop songwriting increasingly valuable in a competitive industry.
Major labels and indie artists alike are exploring how how pop artists use AI tools for hit song creation. Some use these technologies to generate initial ideas, while others employ AI-composed music for specific elements like toplines or backing tracks. The integration of AI music generation into pop workflows continues to evolve as the technology improves.
Finding the right balance between AI music by genre assistance and human creativity remains a key challenge in pop music. Artists want to maintain their unique voice while benefiting from the efficiency and inspiration these tools can provide. This negotiation defines how AI in pop music is being integrated into the mainstream industry.
USC’s AI tool analyzed “Old Town Road” by Lil Nas X and demonstrated how artificial intelligence in music genres works in genre classification. The system identified country elements in the lyrics, rock influences in the chord structure, and pop characteristics in the overall sound. This analysis shows how AI music tools can help understand genre-bending hits that define modern pop.
AI in Classical and Orchestral Music
AI in classical music faces a unique set of challenges compared to modern genres. Classical compositions demand precision in structure, harmony, and orchestration that stretches what AI classical composition systems can currently achieve.
When creating orchestral arrangements, AI must understand not just basic music theory but also how dozens of different instruments work together. These AI music tools need to know that flutes sound different in their upper register than their lower one, or that string sections create different textures depending on how they’re played. This deep knowledge of instrumental capabilities makes AI in classical music one of the most technically demanding areas of music genre transformation through AI.
AI completing unfinished works by classical masters has become one of the most talked-about applications. By studying Mozart’s or Schubert’s complete works, AI classical composition programs can generate music that continues their specific style. This raises important questions about authenticity and artistic legacy, especially in classical music where a composer’s unique voice is so highly valued.
The reception of can artificial intelligence compose authentic classical music varies widely within traditional classical communities. Some see it as a fascinating technical achievement and useful compositional tool, while others question whether machine-created works can ever capture the depth and emotion associated with human-composed classical music.
These discussions about AI music by genre often center on whether emotional expression – considered central to classical music – can be algorithmically generated or if it requires human experience and intention. This philosophical question underlies much of the debate about AI in classical music.
Beethoven’s 10th Symphony completion project where AI finished the work based on his incomplete sketches demonstrates the potential of AI classical composition. The project combined machine learning music analysis with human musicologists to create a plausible completion of Beethoven’s final, unfinished symphony, showing how AI-composed music can work within established classical traditions.
AI in Ambient and Experimental Music
AI in ambient and experimental music represents perhaps the most natural fit between artificial intelligence and musical genre. The focus on texture, atmosphere, and gradual evolution aligns perfectly with what AI ambient music generation systems do well.
AI creates ambient music that constantly shifts and evolves, never repeating exactly the same pattern twice. These AI music tools produce endless streams of atmospheric sounds that gradually transform over time, perfect for situations where obvious loops would break the immersion. That’s why AI in ambient music works so well for meditation apps, art installations, and movie soundtracks – places where the music needs to maintain a consistent mood without calling attention to itself through repetition.
Sound design and textural composition benefit greatly from AI ambient music generation capabilities. Systems can create complex, layered sounds that would take humans hours to design manually. These tools allow composers to quickly generate and explore sonic possibilities that might otherwise remain undiscovered.
Experimental musicians are using the future of AI in experimental and ambient music to push creative boundaries in ways that challenge traditional composition. By creating systems that generate unexpected sounds and combinations, artists can discover new sonic territories and compositional approaches. This experimental application of AI music by genre tools drives innovation in both music and technology.
The use of AI-composed music in installation art and multimedia experiences has grown significantly. Galleries, public spaces, and virtual environments increasingly feature ambient compositions that adapt and evolve in response to various inputs. This integration of AI in ambient music with physical and digital spaces creates new possibilities for immersive art experiences.
Brian Eno’s collaboration with AI systems demonstrates how established ambient musicians are embracing these technologies. Eno, a pioneer of ambient music, has explored how AI ambient music generation can extend his compositional approach, showing how even genre innovators find value in these new tools.
AI in Rock and Alternative Music
AI in rock music faces particular challenges in capturing the raw energy and attitude that defines the genre. The rebellious spirit and emotional intensity of rock often seem at odds with algorithmic creation, making this an interesting test case for AI music by genre technologies.
Simulating authentic guitar techniques and band dynamics poses specific technical hurdles for AI music tools in rock contexts. Programming convincing distorted guitar solos, drum fills with human-like feel, and the natural interplay between band members requires sophisticated modeling of both instruments and performance styles. These challenges make AI in rock music a complex but fascinating area of development.
Despite these difficulties, AI-generated drum patterns and rhythm section components are finding their way into rock production. Some producers use these elements as starting points or backing tracks, allowing them to focus on the more expressive aspects of rock performance. This selective application of AI music generation preserves the human element while benefiting from technological assistance.
Rock artists incorporating AI face the particular challenge of maintaining authenticity. In a genre where authenticity is highly valued, artists must carefully consider how to use AI-composed music without compromising their connection with audiences. This balancing act defines how AI in rock music is evolving and being integrated into creative workflows.
AI analysis of classic rock recordings shows how these systems can identify characteristic elements of different rock subgenres and artists. This application of machine learning music analysis helps understand what makes rock music sound authentic, informing both AI development and human composition in the genre.
AI in Jazz and Improvisational Styles
AI in jazz music represents perhaps the greatest challenge for artificial intelligence in music creation. The genre’s emphasis on improvisation, complex harmony, and interactive performance makes it particularly difficult for algorithms to master.
Teaching AI to understand jazz improvisation requires sophisticated modeling of musical conversation and response. These AI music tools must learn not just scales and chords, but also how to respond contextually to other musical elements in real-time. This makes AI in jazz music one of the most advanced applications of music genre transformation through AI.
Real-time AI systems that can jam with human musicians are emerging as particularly valuable tools. These applications can listen to what a human plays and respond with appropriate musical ideas, creating a back-and-forth that mimics jazz improvisation. Such interactive AI music by genre systems blur the line between tool and collaborator.
Interpreting the complex harmonic structures common in jazz presents specific technical challenges. Advanced AI jazz composition systems must understand extended chords, substitutions, and modal concepts that go beyond the simpler harmonic frameworks of many popular genres. This deep musical knowledge is essential for creating convincing AI-composed music in the jazz tradition.
The balance between structure and spontaneity remains a fundamental question in AI in jazz music. While AI can learn jazz standards and theory, capturing the spontaneous creativity that defines great jazz performance remains elusive. This tension makes jazz an important testing ground for the creative limits of artificial intelligence in music genres.
Improvisational AI systems like Continuator demonstrate how AI jazz composition can work in interactive settings. These tools respond to human musicians in real-time, creating musical dialogues that blend human and machine creativity in new ways.
Genre-Specific AI Adaptation Patterns
What makes AI music by genre particularly fascinating is how the same underlying AI tools produce dramatically different results across musical styles. This variation reveals both the flexibility of these technologies and the distinct characteristics of different genres.
The technical requirements for AI to authentically reproduce different genres vary significantly. An AI music generation system that excels at electronic dance music might fail completely when attempting classical composition, not because the AI itself changes, but because the musical languages are so different. These differences highlight the unique attributes that define each genre.
Underlying mathematical adjustments needed for genre credibility often involve fine-tuning how systems handle rhythm, harmony, and timbre. For example, artificial intelligence in music genres like blues requires specific attention to microtiming and note bending that would be inappropriate in classical music. These adjustments demonstrate how deeply genre conventions are embedded in musical expectations.
Training data composition dramatically affects how AI music by genre systems perform across different styles. An AI trained primarily on rock music will struggle to create convincing jazz, while one trained on a broader musical dataset might produce more generic results across all genres. This relationship between training data and output quality shows how specialized AI music tools must become to excel in specific genres.
Cross-genre analysis tools help demonstrate these adaptation patterns by showing how the same musical elements are treated differently across styles. These comparative analyses improve our understanding of both machine learning music analysis and the defining characteristics of different musical traditions.
AI-Powered Genre Fusion and New Genre Creation
One of the most exciting applications of AI music by genre technology is its ability to blend traditionally separate musical styles. By training on multiple genres simultaneously, AI can create hybrid forms that combine elements in ways human composers might not consider.
AI tools break down walls between music styles in ways humans rarely try. These systems don’t have the same cultural baggage and genre loyalties that human musicians develop, so they freely combine elements from different traditions. This freedom lets AI blend orchestral sounds with electronic beats or mix folk melodies with hip-hop production in ways that feel fresh rather than forced. Many successful projects have shown how these combinations can create interesting music that doesn’t fit neatly into existing categories.
The potential for entirely new genres emerging from AI experimentation is significant. Just as electronic music spawned countless subgenres through technological innovation, AI music by genre systems may create entirely new musical categories that eventually develop their own communities and conventions. This generative potential makes AI an important force in musical evolution.
AI platforms designed for cross-genre experimentation provide specific tools for this kind of musical hybridization. These systems allow users to blend genre-specific elements in customizable ways, accelerating the process of musical fusion and innovation.
The Business Impact of AI Across Music Genres
The commercial adoption of AI music by genre varies significantly across different musical communities. Electronic, pop, and film music businesses have embraced these technologies most quickly, while traditional genres like jazz and classical show more measured integration of AI music tools.
Money-making approaches for AI music differ greatly depending on the genre. In commercial styles like pop and advertising music, AI often creates finished products that clients can use immediately. In artistic genres like jazz or classical, AI usually serves as a tool that human composers use to expand their ideas rather than replace their work. These differences change how people value and pay for AI music across different markets. Record companies have very different strategies for using these technologies too.
Major labels invest in proprietary systems to maintain competitive advantage, while indie labels often leverage publicly available tools to level the playing field. This varied adoption creates an uneven landscape of AI-assisted music production across the industry.
Independent artists using AI tools to compete with larger labels demonstrate how these technologies can democratize music production across genres. These success stories highlight the potential of AI music generation to reshape industry power dynamics.
AI Music Marketing and Distribution by Genre
AI music by genre technologies are transforming not just how music is created, but also how it’s marketed and distributed. Genre-specific optimization strategies help artists reach their target audiences more effectively across streaming platforms.
AI systems now optimize music SEO across different genres by analyzing what musical and metadata elements perform best in each category. This allows artists to tailor their AI-composed music for maximum visibility within their specific genre markets. Such targeted approaches improve discovery in an increasingly crowded streaming landscape.
Promotional strategies for AI-created music vary significantly by genre. Electronic music audiences generally respond well to the technological aspects of AI creation, while rock fans might be more skeptical of machine involvement. These differences require genre-specific messaging around artificial intelligence in music genres to effectively reach different communities.
Streaming platforms categorize and recommend AI music differently across genres. Some platforms have created specific playlists for AI-composed works, while others integrate them into existing genre categories. How these platforms handle AI music by genre significantly impacts artist visibility and audience reception.
Audience reception to AI music varies dramatically across different genre communities. Electronic music fans typically show greater openness to AI music generation, while jazz and rock audiences often express more concern about authenticity and human expression. These reception patterns influence how AI music is marketed and positioned within each genre ecosystem.
AI tools helping independent musicians optimize their SEO by genre demonstrate the growing importance of these technologies in music marketing. These platforms analyze genre-specific trends and listener behavior to help artists position their music effectively, showing how AI music tools extend beyond creation into promotion and distribution.
Ethical Considerations Across Musical Traditions
Concerns about AI authorship vary significantly across different musical communities. Classical and jazz traditions that highly value individual artistic voice often express greater concern about AI-composed music than electronic genres where technology and music have always been closely linked. These varied perspectives reflect deeper cultural values associated with different musical traditions.
The cultural impact of AI music by genre technologies differs between traditional and modern musical forms. Genres with centuries of human tradition may view AI as more disruptive or concerning than recently established styles that emerged alongside digital technology. These differences highlight the varied relationships between technology and tradition across musical cultures.
Questions of appropriation arise when AI recreates culturally specific genres. When systems trained on traditional music from specific cultures generate new works in those styles, complex questions emerge about ownership, respect, and cultural context. These ethical dimensions of artificial intelligence in music genres require careful consideration by developers and users alike.
Different genre communities are responding to AI integration in varied ways. Some embrace the technology as an extension of their creative toolkit, while others establish boundaries to preserve what they see as essential human elements of their tradition. These responses shape how AI music by genre continues to develop across different musical spaces.
Initiatives to establish ethical guidelines for AI music creation are popping up as more people recognize the complicated questions AI raises. These efforts try to create some basic rules that make sense for different musical communities rather than using one-size-fits-all solutions. They recognize that what works for electronic music might feel deeply disrespectful in traditional folk music from specific cultures.
Future Predictions: AI’s Evolution in Music Genres
Tomorrow’s AI music by genre tools won’t just get better—they’ll get more specialized. Instead of general music generators, we’ll see AI that truly understands the difference between West Coast hip-hop and East Coast hip-hop, or between Chicago blues and Delta blues. These systems will pick up on the subtle details that genre fans immediately recognize, creating music that sounds less generic and more rooted in specific traditions.
Some genres will likely continue to embrace AI more readily than others. Electronic, pop, and film music will probably see the deepest integration of AI music generation, while genres that particularly value human performance aspects may maintain more selective adoption of these tools.
Human musicians across genres will adapt their roles as AI music tools become more capable. Many will likely position themselves as curators and directors of AI systems rather than competitors with them. This shift will redefine the creative process across different musical traditions while maintaining the essential human element in music creation.
The long-term impact on musical diversity and creativity remains an open question. Will AI music by genre lead to greater homogenization as systems learn from the same datasets? Or will it enable more experimentation and novel combinations? The answer will likely vary across different musical communities and depend on how these technologies are implemented and guided.
Emerging applications like real-time collaborative AI jamming systems point to exciting new possibilities for artificial intelligence in music genres. These tools could create entirely new performance paradigms and creative workflows that blend human and machine contributions in previously impossible ways.
Frequently Asked Questions About AI in Music Genres
How does AI adapt differently to create music in various genres?
AI music by genre systems adapt through specialized training on genre-specific datasets and customized algorithms. An AI creating classical music uses different parameters and approaches than one making hip-hop, just as human composers would.
Which music genre has seen the most successful AI integration so far?
Electronic dance music has generally seen the most successful integration of AI music generation, largely because its digital nature and pattern-based structure align well with AI capabilities.
Can AI truly understand the cultural context behind different music styles?
Current AI music tools can recognize and reproduce the technical aspects of different genres, but their understanding of cultural context remains limited. They can mimic stylistic elements without truly comprehending their cultural significance.
Will certain genres be more affected by AI than others?
Digital genres with clear patterns will see AI take a bigger role faster. EDM and pop music already use lots of computer tools, so adding AI feels like a small step. But try getting AI to capture the feeling of a jazz quartet that’s been playing together for decades or the raw emotion of a punk band, and you’ll see where machines still fall short.
How can musicians use AI tools specific to their genre?
Musicians can use genre-specific AI music tools for idea generation, backing tracks, arrangement assistance, and production support. The best approach varies by genre, with different tools optimized for different musical styles.
What makes an AI-generated classical piece different from AI-generated EDM?
The differences lie in the training data, musical parameters, and evaluation criteria. Classical AI-composed music must handle complex orchestration and long-form structure, while EDM focuses more on sound design and rhythmic patterns.
Is human creativity still necessary in an era of AI music composition?
Absolutely. AI music by genre tools work best as collaborative partners that extend human creativity rather than replace it. Human direction, curation, and emotional intent remain essential to meaningful musical creation.
How are music streaming platforms handling AI-generated content across genres?
Platforms are developing various approaches to artificial intelligence in music genres, from specific AI music categories to integration within existing genre classifications. These policies continue to evolve as the technology and its adoption advance.
Will AI lead to the emergence of entirely new music genres?
Very likely. Just as new technologies historically sparked new musical forms, AI music generation will probably lead to novel genres that blend existing styles or create entirely new approaches to sound organization.
What skills do human musicians need to collaborate effectively with AI?
Musicians need technical understanding of AI music tools, clear creative vision to direct these systems, and adaptability to incorporate AI outputs into their workflow. The specific skills vary somewhat by genre, but these core capabilities apply broadly.
Check out our related articles on AI music creation tools, AI music production in 2025, the impact of AI in music, AI tools for musicians, and AI music technology innovation to learn more about this rapidly developing field.